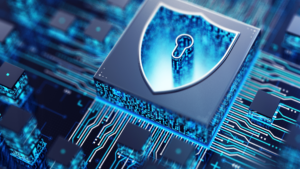
Knowing Your Data
Inevitably, all Continuous Improvement practitioners (especially those leading projects) will come across a set of data related to a specific process or sample being studied. Data analytics is indeed a key part of understanding the voice of the process (VoP) and although there is so much to be learned from advanced analysis, there are three basic and yet extremely important concepts that the CI professional should be comfortable with: 1) central tendency, 2) spread, and consequently, 3) outliers and skewness.
Central Tendency
When looking into central tendency, the analyst is looking for the areas of the dataset (and therefore the process itself) that has more concentration around a certain number, or that show where the true mid-point of the dataset is, or that simply indicates which value(s) appear more often. It is an extremely important statistic since most processes tend to somehow have this concentration of values (hence why it is called central tendency). Graphically, one can use a simple histogram to visually investigate central tendency. The analyst can also calculate statistics such as the mean (or average), the median (the true mid-point of the data), and the mode (the most frequent values).
Spread
Also known as variation, the spread of the data adds valuable insight to central tendency measures. It provides an overview of how far from the concentrated numbers the actual observations are. Think about spread as the measure of variation that prevents values from being nicely concentrated around a certain central tendency measure; for example, the average. The usual statistics to investigate spread are variance and standard deviation.
Outliers & Skewness
Not only the CI professional should understand where most values are concentrated (central tendency) and how much of spread (variation) is found in the data but also, they should investigate where specific values that are so far from the central tendency are located – they’re called outliers and there is a specific way of calculating them. Outliers influence averages and therefore produce something called skewness. Although outliers do not influence the median as much, they should still be investigated when working with median values. Do these values really belong to the process? Is this an expected one-off event? Or has the process really changed?
How Adonis Can Help
Data-driven decision-making is our forte. Here at Adonis, we provide training and ongoing support that will help your organization properly perform robust data analytics that will drive your teams to excel at drawing meaningful insights from data.